A New Report on Medical Debt Research and Identification in Court Records
James Carey | April 23, 2024
There is a medical debt crisis in America, but it is difficult to see due to a lack of data, especially around one of its greatest nexuses: our courts. This report examines the landscape of research on the impoverishing effects of medical debt and the hurdles to data-centered research in the field. Acknowledging that courts are the focus of a large amount of medical debt collection, it explores the challenges in using court data to investigate medical debt collection. One of the largest roadblocks is difficulty in identifying medical debt in court data, and this report evaluates good methods of doing so with low overhead. One potentially successful method was developed for the report and focuses on using expert consultation from a physician to identify plaintiffs.
The report dives into both the challenges of conducting research on medical debt using court records and some effective tools to do so through plaintiff identification. The code used for the case study section of this report can be accessed on the Civil Justice Data Commons GitHub and may prove a useful starting point for future work in this area.
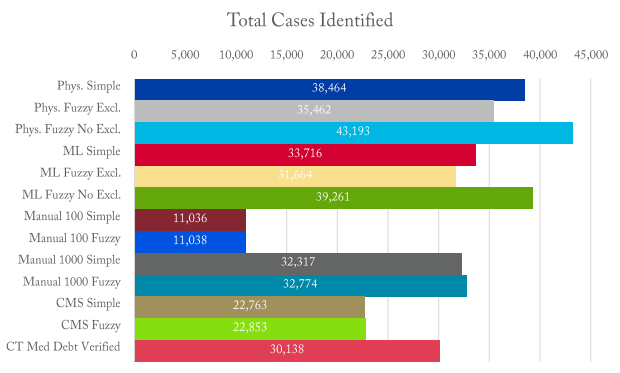
This graph shows a preview of the results found in the report comparing how well different methods identify Med Debt Cases.
The medical expertise of Dr. Fatu S. Conteh was invaluable in developing this method of identification, as were the data policy skills and work of Stephanie Straus and Jordan Rinaldi of the Massive Data Institute, as well as the guidance of Dr. Amy O’Hara of the Massive Data Institute and Professor Tanina Rostain of Georgetown University Law Center.
This report was supported by a grant from the JPB Foundation.